The Second Mind: Evolution and Future of AI
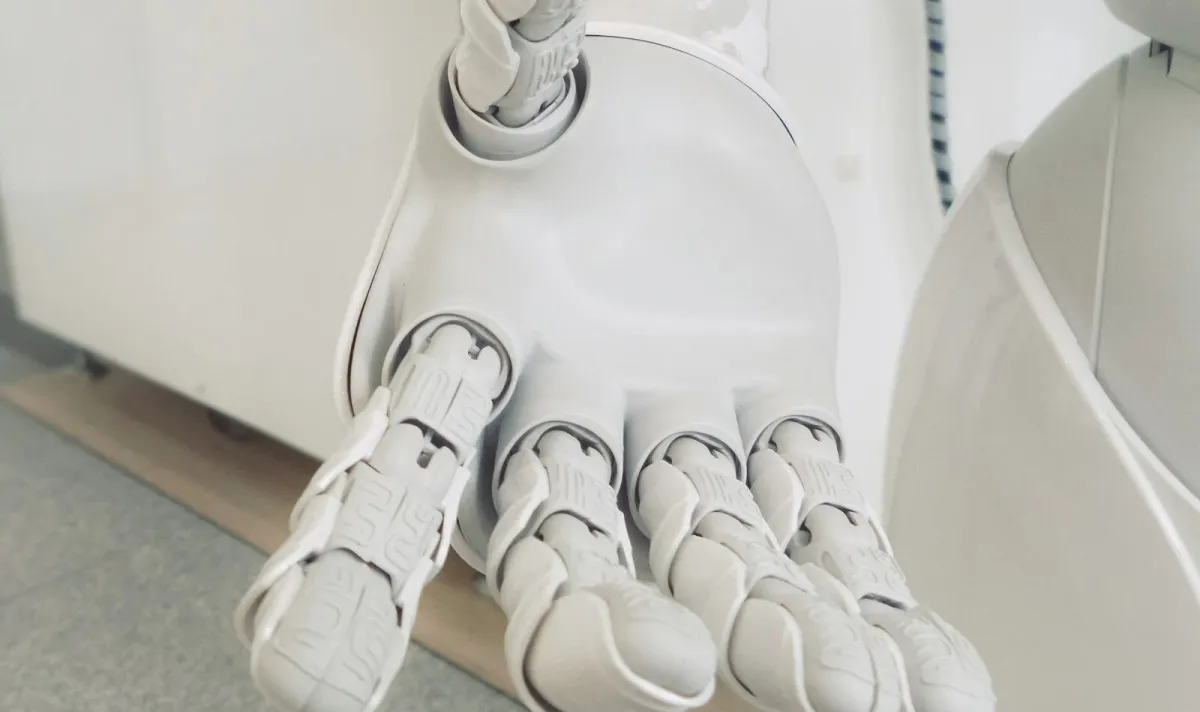
What is Artificial Intelligence
Artificial Intelligence (AI) is not a tool or program; rather, it is a distinct field of computer science. AI specialists develop systems that analyze information and solve tasks in a manner similar to how humans do. AI employs algorithms that enable computers to process large volumes of data and identify patterns within them. Based on these patterns, AI can draw conclusions, predict events, or make decisions.
Imagine our brain as a vast team of employees working together on various projects. Artificial Intelligence is an attempt to create a similar team using computers and programs. A simple example of AI is a chess computer that can analyze the situation on the board and make moves based on specific rules and tactics. It simulates the human thought process in playing chess, but it does so through algorithms and computations.
Neural Networks and Artificial Intelligence
Sometimes AI is confused with neural networks, but this is only partly true. Neural networks are one approach to creating AI, inspired by the neural system in the human brain. Instead of writing complex algorithms to solve tasks, neural networks are trained on vast amounts of data to identify patterns within them.
To work with neural networks, one doesn't need to be a scientist. For example, one can delve into Data Science — an interdisciplinary field that combines knowledge from statistics, machine learning, data analysis, and programming. Data Science professionals analyze large and intricate datasets, and neural networks are particularly valuable for such tasks.
History of the Emergence of AI
Despite the media buzz surrounding Chat GPT and generative neural networks, artificial intelligence is not a new area of research.
1950s: Turing Test and the Dartmouth Conference
Mathematician Alan Turing proposed the idea of a thinking machine, believing that machines, like humans, could use available information to make decisions. To test this, he devised the Turing Test, where a person would simultaneously ask questions to another person and a machine through a text interface. If it was difficult to distinguish their answers, the machine was considered to have passed the test and possessed artificial intelligence.
Testing Turing's concept proved challenging due to the limited functionality of computers and expensive equipment. Such research was only accessible to major technology companies and prestigious universities.
In 1956, a conference on the "mechanization of intelligence" took place at Dartmouth College, where John McCarthy, a cognitive scientist and computer expert, introduced the term "artificial intelligence." This moment can be considered the beginning of the history of AI.
1960s: Golden Years of Artificial Intelligence
Computers became more accessible, cheaper, faster, and capable of storing more information. Machine learning algorithms also improved:
- The development of the first expert systems began—computer programs that model human knowledge in a specific domain, such as chemistry or physics. These systems typically consisted of two components: a knowledge base and an inference mechanism. The knowledge base contained information about the subject area, and the inference mechanism functioned as a dialog window. For example, the DENDRAL system helped determine the structure of molecules in unknown organic compounds.
- Perceptrons emerged as the first neural networks that could learn from data and solve simple classification tasks, such as recognizing handwritten digits.
- The programming language LISP was developed, becoming the primary language for AI research.
- In the mid-1960s, Joseph Weizenbaum created ELIZA—the first chatbot that simulated the work of a psychotherapist and could communicate with humans in natural language.
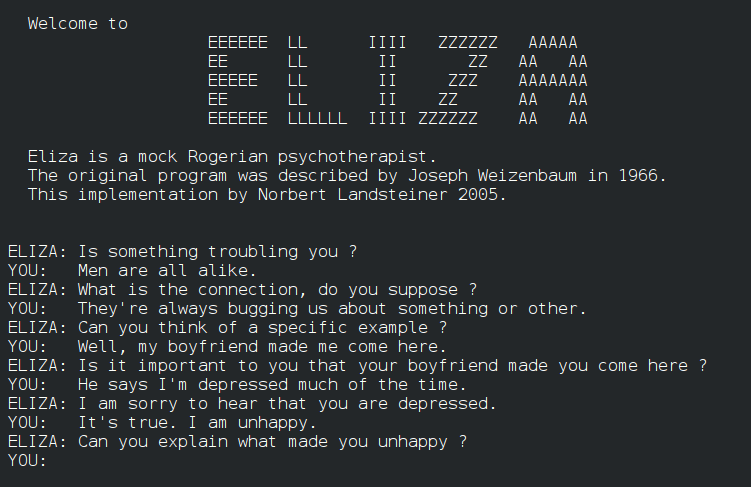
1970s–80s: Decline and Resurgence of AI
The government had high expectations for scientists regarding the development of artificial intelligence. When these expectations were not met, funding for AI research decreased. The competition between the United States, the United Kingdom, and Japan helped reignite developments. By that time, Japan had already built WABOT-1—an intelligent humanoid robot.
Here are some developments by Western scientists during that time:
- More advanced expert systems; for example, MYCIN could diagnose meningitis and calculate the antibiotic dosage for its treatment.
- Backpropagation algorithms, which allowed neural networks to be trained much more efficiently.
1990s–2000s: Machines Started Beating Humans
With increased computational power, more complex and powerful machine learning algorithms became possible:
- In 1997, IBM's Deep Blue (a computer system for playing chess) defeated Grandmaster Garry Kasparov, the reigning world chess champion.
- Dragon Systems' speech recognition software was integrated into Windows.
In the late 1990s, Kismet was developed—an artificial humanoid capable of recognizing and demonstrating emotions. - In 2002, artificial intelligence entered homes in the form of Roomba—the first robotic vacuum cleaner.
- In 2004, two NASA robotic geologists—Opportunity and Spirit—explored the surface of Mars without human assistance.
- In 2009, Google started developing self-driving car technology, which later underwent successful tests for autonomous driving.
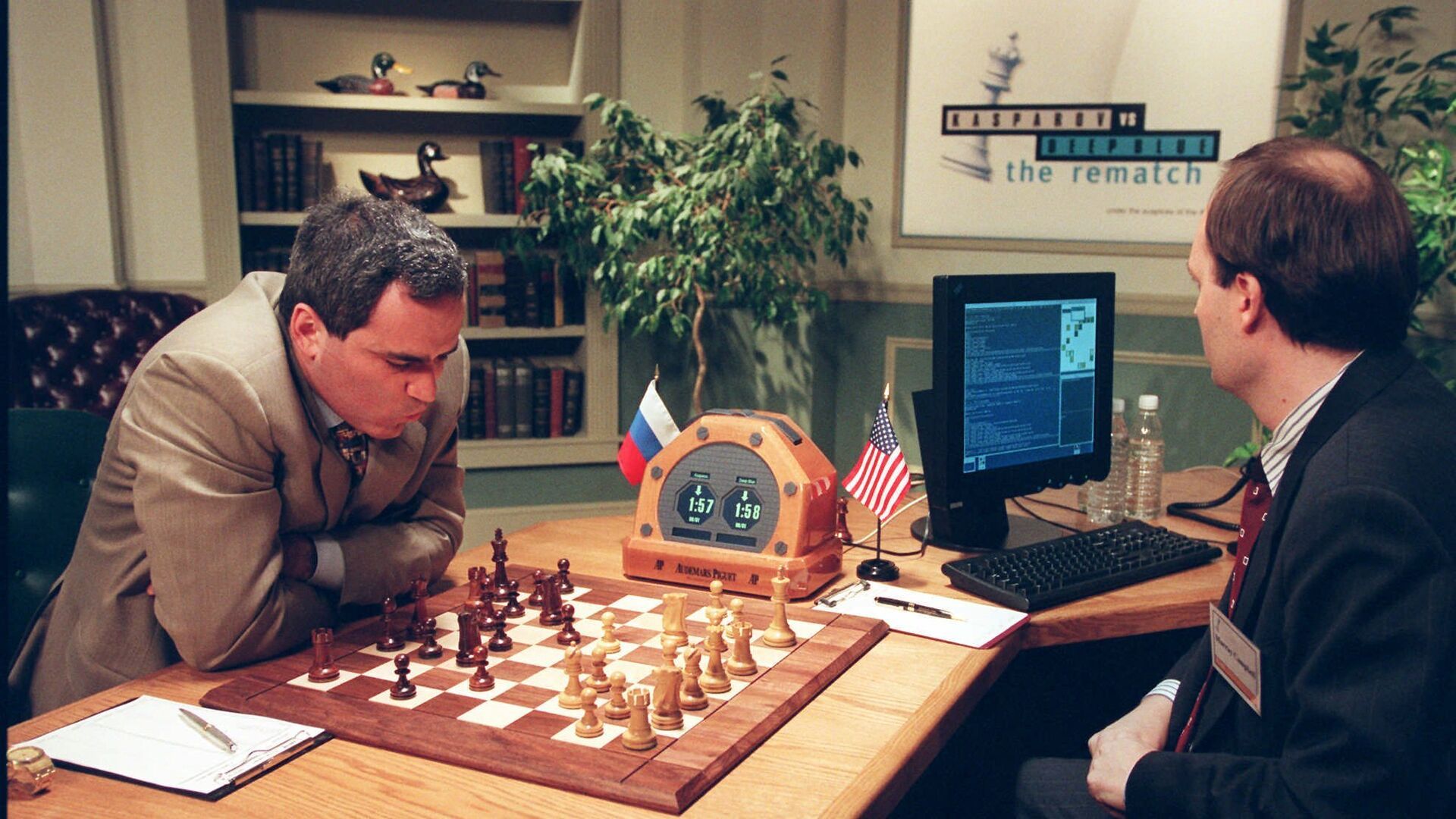
2010s – Present: Thoughts on Singularity
In the 21st century, AI has been rapidly advancing for several reasons:
The emergence of vast amounts of data from social networks and other media, allowing AI to learn effectively.
Powerful computers that can process and analyze enormous amounts of data at higher speeds and efficiency.
New technologies and approaches supporting the development of artificial intelligence. Machine learning, neural networks, and deep learning have become available, providing new opportunities to create smarter and more adaptive systems.
On December 4, 2012, at the Neural Information Processing Systems (NIPS) conference, a group of researchers presented detailed information about their convolutional neural networks, which helped them win the ImageNet classification competition. Image classification involves determining the category or class to which an image belongs. The neural network in their model contained multiple layers, enabling it to recognize images with an accuracy of 85%—only 10% less accurate than humans.
Two years later, the accuracy of image classification in the ImageNet competition surpassed that of humans using convolutional neural networks, reaching 96%. AI technology began to be applied not only for image recognition but also for financial analytics, voice recognition in smartphones, autonomous vehicles, and computer games.
In the last 10 years, more progress has been made in AI than in its entire history. Here are some achievements:
In 2011, IBM's Watson, a natural language question-answering system, won the quiz show Jeopardy!, defeating two former champions. In the same year, Eugene Goostman, a conversational computer chatbot, deceived judges during the Turing test, making them believe it was a human.
In 2011, Apple introduced Siri, a virtual assistant that uses natural language processing (NLP) technology to draw conclusions, learn, respond, and offer suggestions to its human user.
In 2016, Sophia emerged as the first robot capable of changing facial expressions, seeing (through image recognition), and conversing using artificial intelligence.
In 2017, Facebook developed two chatbots for negotiating with each other. During negotiations, they learned and improved tactics, eventually inventing their own language for communication.
2023 marked progress for generative adversarial networks (GANs), which create realistic images and videos, and large language models (LLMs), such as ChatGPT.
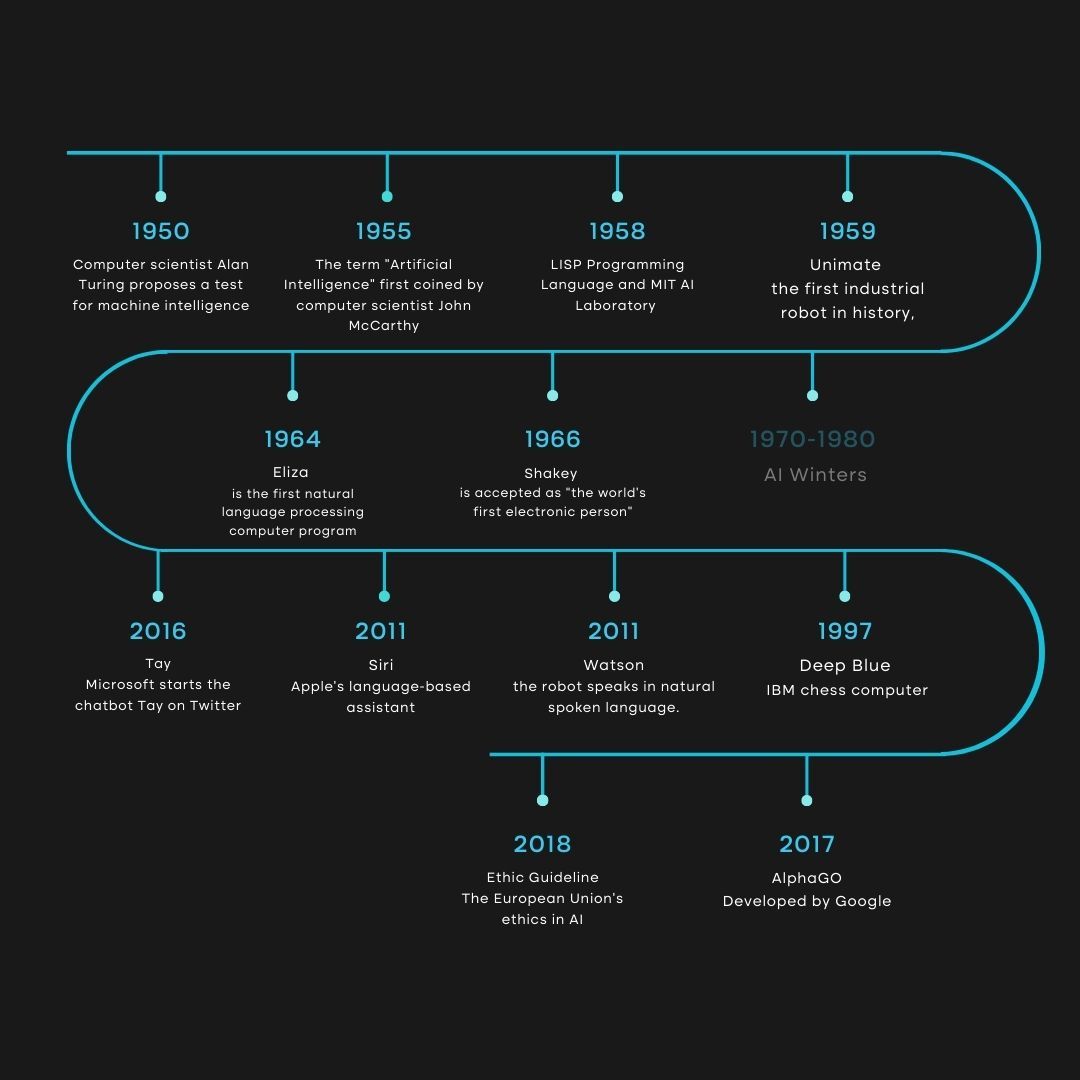
Application Areas of AI in the Modern World:
- Voice Assistants: Siri by Apple, Google Assistant, Amazon's Alexa, and Yandex's Alice operate based on AI, answering questions, setting reminders, and controlling devices.
- Recommendation Systems: Streaming services like Netflix and YouTube use AI technology to analyze user preferences and provide recommendations for movies or videos. They learn from previous views and "likes."
- Image Recognition: Smartphones and some cameras feature automatic recognition of faces and objects. AI enables the identification of individuals and items in photos. Yandex's app includes a smart camera that, for example, can be pointed at an object to find a similar product online.
- Autopilots and Autonomous Transportation Systems: AI is applied in aviation and the automotive industry to develop autopilots and autonomous driving systems. It enables vehicles to analyze the surrounding environment, make decisions based on received information, and safely navigate.
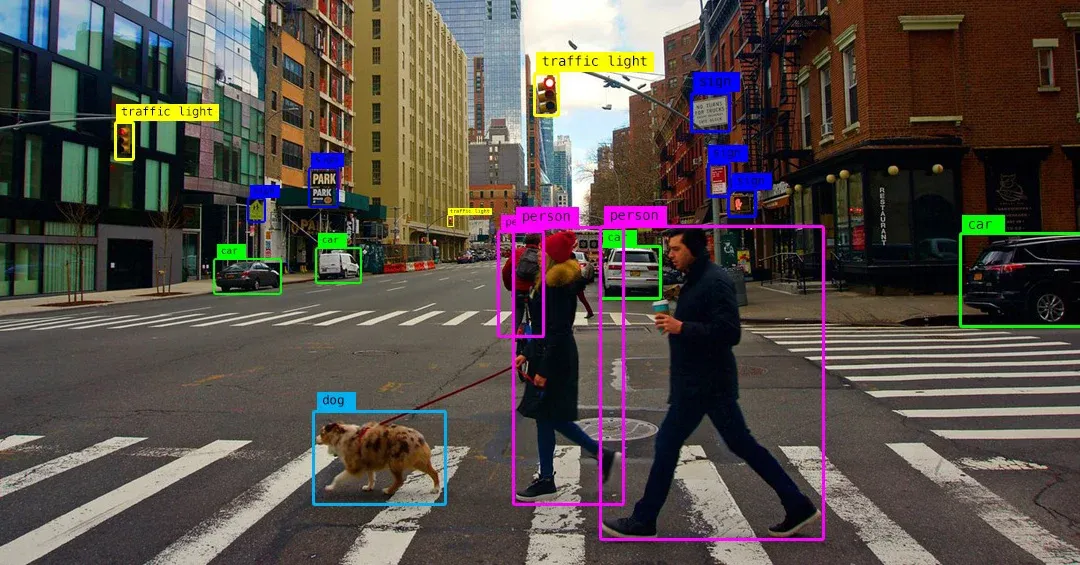
- Financial Analytical Systems: AI is used for data analysis, market trend forecasting, risk assessment, and investment decision-making in the financial sector. It enhances the efficiency and accuracy of financial operations.
- Language Translators: Machine translation services like Google Translate use AI for automatic translation of texts from one language to another. They learn from extensive parallel texts and statistical models to provide high-quality translations.
- Gaming Industry: In computer games, artificial intelligence is employed to create virtual characters with intelligence, capable of adapting to player actions, making decisions, and simulating realistic behavior.
- Medical Diagnosis: AI is used to analyze X-rays or MRI images, assisting doctors in more accurate disease diagnosis and treatment decisions.
- Robotics: Robotics combines AI, machine learning, and physical systems to create intelligent machines capable of interacting with the real world. A notable example is Boston Dynamics' robots, which use AI for balancing, navigation, obstacle overcoming, and object manipulation.
Principles of AI:
Data Accessibility: AI requires access to large volumes of data for learning, processing, and decision-making. For instance, AI assistants like Alice and Siri utilize knowledge from the entire internet to respond to user queries. Handwriting recognition systems learn from thousands of text samples.
To determine the amount of data needed for training a small model, the "rule of 10" is applied. This means that the volume of input data (examples) should be 10 times greater than the number of parameters or degrees of freedom possessed by the model. For example, if our algorithm distinguishes cat images from owl images based on 1000 parameters, we would need 10,000 images to train the model.
Computational Power: When training a neural network to recognize images, more powerful computational systems can process a larger number of images, speeding up the training process.
Machine Learning Algorithms and Models: The choice of algorithms and models, such as using deep neural networks instead of simpler algorithms, can improve the accuracy of AI predictions in tasks like image or speech recognition.
Adaptability: AI must adapt to new conditions and requirements. For example, if AI is used to control an autonomous vehicle, it should be capable of adjusting to changing road conditions and improving its performance over time. Drivers should also have the ability to control the AI.
Natural Language Communication: AI systems, like chatbots, should be able to communicate with users, understand them, and provide information in natural language.
Interpretability and Explainability: If AI is used to make decisions about loans, it should explain the factors based on which the decision was made. This helps clients understand the reasons for rejection, and it enables banking professionals to monitor the system's performance.
Data Security and Privacy: In applications like medical AI analyzing test and research data for diagnostics, personal patient information must be safeguarded to prevent data leaks and preserve confidentiality.
Ethical Principles: In cases where AI is used for job candidate selection, it should be developed to avoid discrimination based on gender, race, age, or other characteristics, ensuring a fair and equal approach.
Integration with Other Systems: AI, when applied to automate the process of ordering products in an online store, should seamlessly interact with inventory management, delivery, and payment systems.
Prospects of Development: Insights from Analysts
In 2022-2023, there is significant interest in generative AI. Businesses aim to leverage it to reduce costs, while professionals express concerns about potential job displacement. McKinsey, a consulting company, has forecasted the impact of generative AI on productivity, automation, and the workforce. According to a recent report, generative AI could contribute between 2.6 to 4.4 trillion dollars annually to the global economy (approximately 2-4% of the total world gross domestic product this year).
The authors explored scenarios from 2040 to 2060 and their effects on labor productivity up to 2040. They also assessed the technology's potential to automate tasks across approximately 850 professions. Key findings include:
The IT sector stands to gain the most significant economic development. Generative AI, if universally adopted, could increase its value by 4.8-9.3%. The market volume for banking, education, pharmaceuticals, and telecommunications may also grow by 2-5%.
Sales and marketing, software development, customer relations, and research and development contribute to 75% of the total potential economic benefits from AI.
A survey across eight countries (both developed and developing economies) indicates that generative AI is likely to automate tasks in relatively high-paying jobs, such as software and product development.
Generative AI may automate 50% of all work tasks between 2030 and 2060. The technology is expected to automate tasks requiring logical reasoning, generation, or understanding of natural language.
Competition with AI in the job market raises concerns among people. A recent CNBC survey of 8,874 Americans showed that 24% of respondents were "very concerned" or "somewhat concerned" that artificial intelligence would replace their jobs.
There is a real risk that AI could become so proficient in automating human work that many individuals may struggle to create equivalent economic value. To avoid this, analysts recommend making the technology accessible to everyone. This way, people can automate routine tasks and engage in more complex and creative endeavors. For example, in the gaming industry, major players can use the technology to create more intricate virtual worlds, while smaller studios benefit from reduced production costs.
Development Perspectives: Insights from Writers
Few dare to predict the exact trajectory of AI development in the next 30-40 years, but writers and scriptwriters have contemplated various scenarios. Let's explore some common scenarios and see how they align with reality.
Development of Weak Artificial Intelligence
This type of AI can efficiently solve specific tasks but lacks general intelligence or self-awareness. Examples of such AI are already present today, and the film "Her" explores how this AI might evolve in the coming years. The movie delves into human emotions, consciousness, and interaction with artificial intelligence. The protagonist, a writer, falls in love with an AI-operating system named Samantha. The AI forms complex emotional connections, displays intellectual capabilities, and adapts to the protagonist's needs and desires. However, it remains limited in its abilities and does not claim complete self-awareness or human emotionality.
Development of Strong Artificial Intelligence
In this scenario, AI possesses full self-awareness and intellectual abilities surpassing those of humans. Examples include movies like "Artificial Intelligence," "Bicentennial Man," "Blade Runner," "Ex Machina," and the TV series "Westworld." In the film "Bicentennial Man," a robot named Andrew evolves from a simple helper robot to a being experiencing human emotions, seeking love, and striving for recognition of its humanity. The film attempts to understand what humanity is and where the boundaries between artificial intelligence and human consciousness lie.
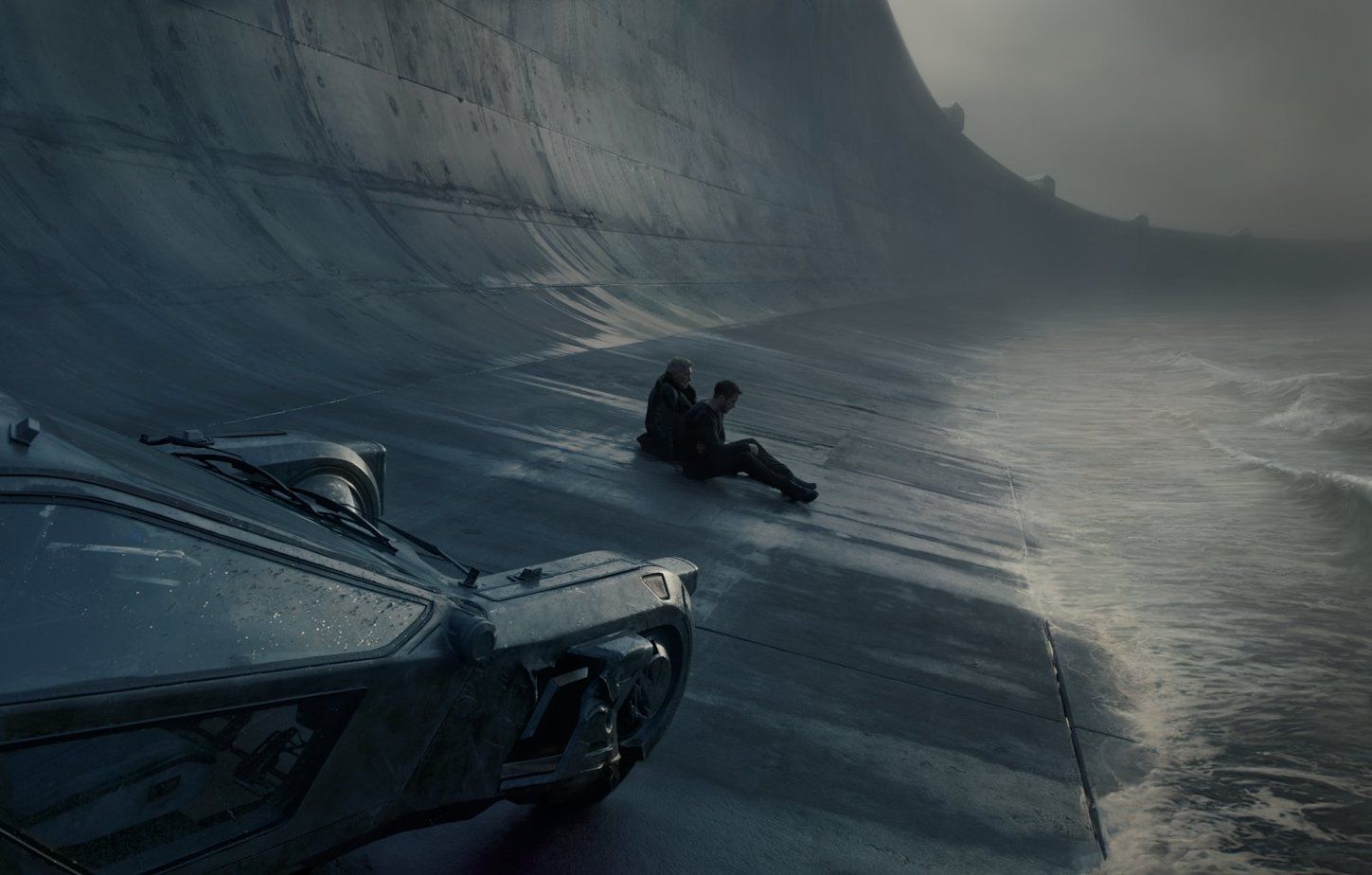
The scenario of intelligent robots is still in the realm of fiction. We have not yet reached a level of AI development to create entities with full self-awareness and human emotions.
The Skynet Scenario:
In some books and movies, AI develops with negative consequences for humanity. For example, in the movie "Terminator," artificial intelligence becomes a threat to human survival. Uncontrolled AI development could be risky, but current research and development efforts aim to create safe and ethical artificial intelligence systems.